Machine Learning
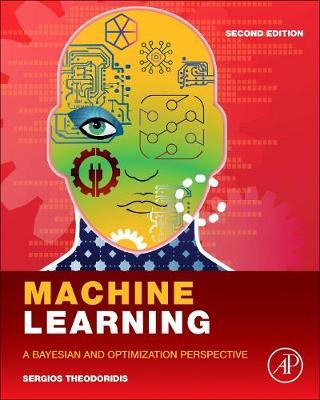
Machine Learning
This palette of techniques concludes with an extended chapter on neural networks and deep learning architectures. The book also covers the fundamentals of statistical parameter estimation, Wiener and Kalman filtering, convexity and convex optimization, including a chapter on stochastic approximation and the gradient descent family of algorithms, presenting related online learning techniques as well as concepts and algorithmic versions for distributed optimization.
Focusing on the physical reasoning behind the mathematics, without sacrificing rigor, all the various methods and techniques are explained in depth, supported by examples and problems, giving an invaluable resource to the student and researcher for understanding and applying machine learning concepts. Most of the chapters include typical case studies and computer exercises, both in MATLAB and Python.
The chapters are written to be as self-contained as possible, making the text suitable for different courses: pattern recognition, statistical/adaptive signal processing, statistical/Bayesian learning, as well as courses on sparse modeling, deep learning, and probabilistic graphical models.
New to this edition:
Complete re-write of the chapter on Neural Networks and Deep Learning to reflect the latest advances since the 1st edition. The c
PRP: 688.08 Lei
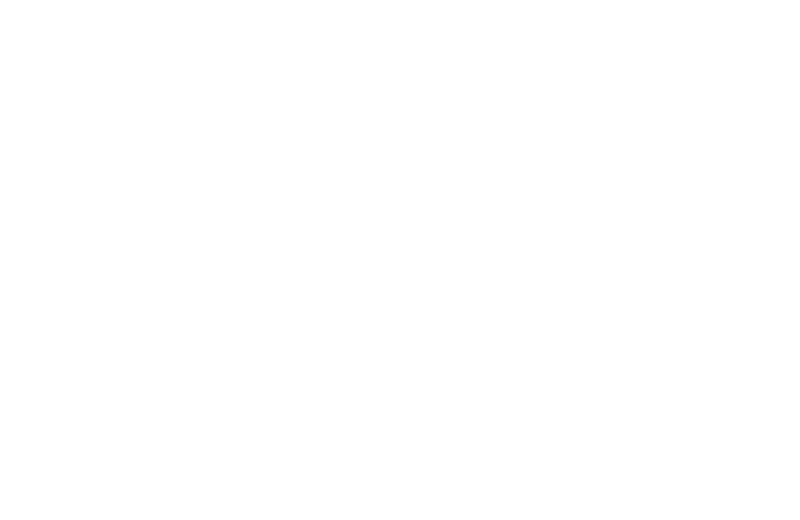
Acesta este Pretul Recomandat de Producator. Pretul de vanzare al produsului este afisat mai jos.
584.87Lei
584.87Lei
688.08 LeiLivrare in 2-4 saptamani
Descrierea produsului
This palette of techniques concludes with an extended chapter on neural networks and deep learning architectures. The book also covers the fundamentals of statistical parameter estimation, Wiener and Kalman filtering, convexity and convex optimization, including a chapter on stochastic approximation and the gradient descent family of algorithms, presenting related online learning techniques as well as concepts and algorithmic versions for distributed optimization.
Focusing on the physical reasoning behind the mathematics, without sacrificing rigor, all the various methods and techniques are explained in depth, supported by examples and problems, giving an invaluable resource to the student and researcher for understanding and applying machine learning concepts. Most of the chapters include typical case studies and computer exercises, both in MATLAB and Python.
The chapters are written to be as self-contained as possible, making the text suitable for different courses: pattern recognition, statistical/adaptive signal processing, statistical/Bayesian learning, as well as courses on sparse modeling, deep learning, and probabilistic graphical models.
New to this edition:
Complete re-write of the chapter on Neural Networks and Deep Learning to reflect the latest advances since the 1st edition. The c
Detaliile produsului