Graph Representation Learning
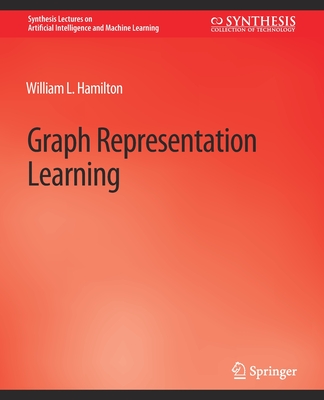
Graph Representation Learning
Graph-structured data is ubiquitous throughout the natural and social sciences, from telecommunication networks to quantum chemistry. Building relational inductive biases into deep learning architectures is crucial for creating systems that can learn, reason, and generalize from this kind of data. Recent years have seen a surge in research on graph representation learning, including techniques for deep graph embeddings, generalizations of convolutional neural networks to graph-structured data, and neural message-passing approaches inspired by belief propagation. These advances in graph representation learning have led to new state-of-the-art results in numerous domains, including chemical synthesis, 3D vision, recommender systems, question answering, and social network analysis.
This book provides a synthesis and overview of graph representation learning. It begins with a discussion of the goals of graph representation learning as well as key methodological foundations in graph theory and network analysis. Following this, the book introduces and reviews methods for learning node embeddings, including random-walk-based methods and applications to knowledge graphs. It then provides a technical synthesis and introduction to the highly successful graph neural network (GNN) formalism, which has become a dominant and fast-growing paradigm for deep learning with graph data. The book concludes with a synthesis of recent advancements in deep generative models for graphs--a nascent but quickly growing subset of graph representation learning.
PRP: 557.91 Lei
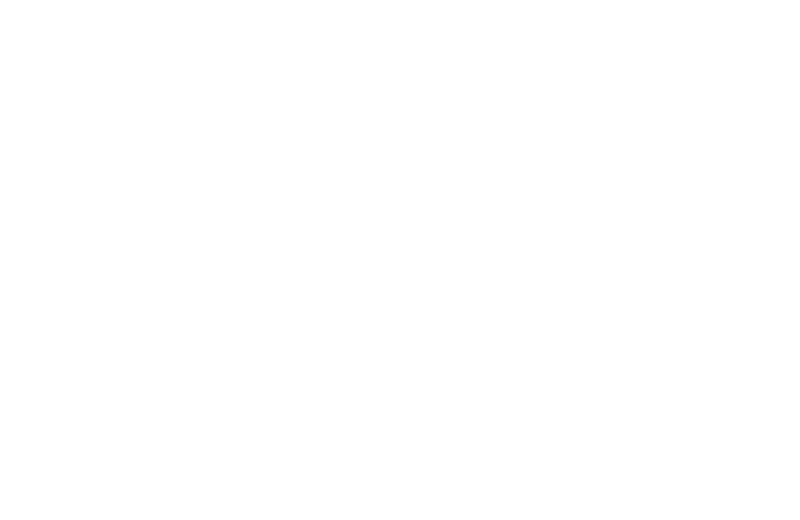
Acesta este Pretul Recomandat de Producator. Pretul de vanzare al produsului este afisat mai jos.
502.12Lei
502.12Lei
557.91 LeiLivrare in 2-4 saptamani
Descrierea produsului
Graph-structured data is ubiquitous throughout the natural and social sciences, from telecommunication networks to quantum chemistry. Building relational inductive biases into deep learning architectures is crucial for creating systems that can learn, reason, and generalize from this kind of data. Recent years have seen a surge in research on graph representation learning, including techniques for deep graph embeddings, generalizations of convolutional neural networks to graph-structured data, and neural message-passing approaches inspired by belief propagation. These advances in graph representation learning have led to new state-of-the-art results in numerous domains, including chemical synthesis, 3D vision, recommender systems, question answering, and social network analysis.
This book provides a synthesis and overview of graph representation learning. It begins with a discussion of the goals of graph representation learning as well as key methodological foundations in graph theory and network analysis. Following this, the book introduces and reviews methods for learning node embeddings, including random-walk-based methods and applications to knowledge graphs. It then provides a technical synthesis and introduction to the highly successful graph neural network (GNN) formalism, which has become a dominant and fast-growing paradigm for deep learning with graph data. The book concludes with a synthesis of recent advancements in deep generative models for graphs--a nascent but quickly growing subset of graph representation learning.
Detaliile produsului